Arturo Deza, Devi Parikh, “Understanding Image Virality”, Proceedings of the IEEE Conference on Computer Vision and Pattern Recognition (CVPR), 2015, pp. 1818-1826.
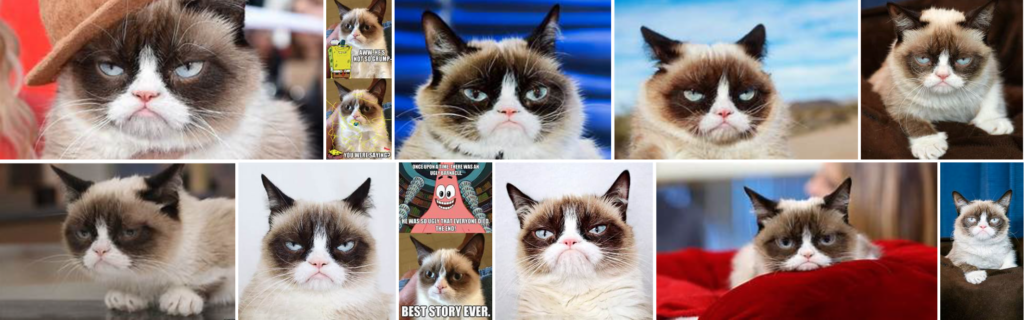
Abstract: (…) In this paper we study viral images from a computer vision perspective. We introduce three new image datasets from Reddit, and define a virality score using Reddit metadata. We train classifiers with state-of-the-art image features to predict virality of individual images, relative virality in pairs of images, and the dominant topic of a viral image. We also compare machine performance to human performance on these tasks. We find that computers perform poorly with low level features, and high level information is critical for predicting virality. We encode semantic information through relative attributes. We identify the 5 key visual attributes that correlate with virality. We create an attribute-based characterization of images that can predict relative virality with 68.10% accuracy (SVM+Deep Relative Attributes) — better than humans at 60.12%. Finally, we study how human prediction of image virality varies with different ‘contexts’ in which the images are viewed, such as the influence of neighbouring images, images recently viewed, as well as the image title or caption (…). Read more